The Best AI Data Analytics Tools (in 2025)
We tested over 150 analytics platforms and selected the best tools to process, analyze, and unlock insights from your data using advanced AI techniques.
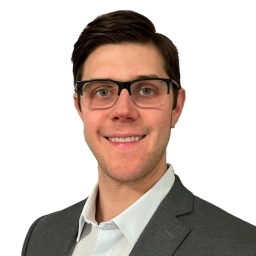
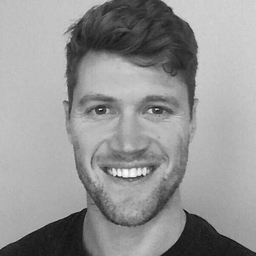
Data analysts and business teams are drowning in spreadsheets, dashboards, and manual data wrangling that eats up countless hours and still leaves critical insights buried. Modern AI data analytics tools are transforming this landscape by automating tedious tasks, surfacing hidden patterns instantly, and making advanced analytics accessible to everyone from data scientists to business users.
After extensively testing dozens of AI-powered analytics platforms over the past month, we've identified the most powerful and user-friendly tools that are revolutionizing how organizations extract value from their data in 2025.
Quick look: The 8 best AI Data Analytics Tools
- ThoughtSpot Best for searching your data instantlySee Tool
- Snorkel AI Best for automating data labelingSee Tool
What makes the best AI Data Analytics Tools?
Here's what our evaluation focused on to identify the most effective AI data analytics tools:
- Automation: Advanced AI capabilities automate complex data preparation, cleaning, and analysis tasks. The best tools significantly reduce manual work through smart suggestions and automated workflows that adapt to different data types.
- Integration: Connecting seamlessly with existing data sources and tools determines real-world utility. Top platforms offer extensive native connectors to databases, cloud services, and business applications while maintaining consistent performance.
- Collaboration: Modern analytics requires seamless teamwork between technical and business users. Leading tools enable real-time collaboration through shared workspaces, version control, and role-based access that keeps projects organized.
- User Experience: An accessible interface empowers users across technical skill levels. The most effective platforms strike the right balance between powerful capabilities and ease of use, with clear documentation and intuitive workflows.
The best AI Data Analytics Tools at a glance
Tool | Best For | Standout Features | Pricing |
---|---|---|---|
Alteryx | Automating Data Prep | Code-free workflow automation canvas | Enterprise pricing requires quote request |
ThoughtSpot | Searching Your Data Instantly | Natural language search for data. | Enterprise pricing requires contacting sales. |
Akkio | Building Business Predictions | No-code predictive model building. | Pricing tailored for SMB needs. |
Snorkel AI | Automating Data Labeling | Programmatic training data labeling. | Enterprise pricing requires significant investment. |
Ataccama | Automating Data Management | Unified data quality and governance. | Enterprise pricing requires contacting sales. |
Dataiku | Driving Enterprise AI Collaboration | Unified code/no-code collaboration. | Custom enterprise pricing plans available. |
Hex | Collaborating on Analytics Notebooks | Share notebooks as interactive apps. | Free tier available; enterprise plans costly. |
People.ai | Unlocking Revenue Intelligence | Automated sales activity data capture. | Enterprise pricing for larger sales teams. |
Best AI Data Analytics Tool for Driving Enterprise AI Collaboration
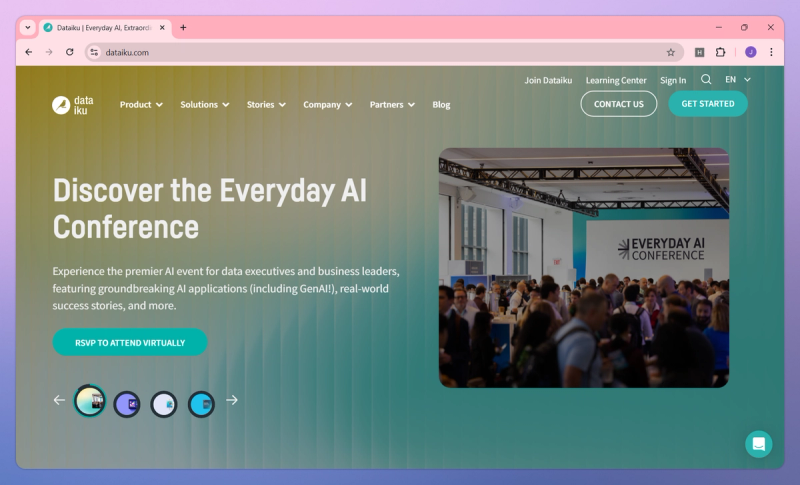
Dataiku
Dataiku is an end-to-end data science platform that enables teams to build, deploy, and manage AI projects collaboratively. Its core value lies in democratizing data workflows – technical coders and non-coders alike can easily prepare data, create ML models, and visualize results on one platform. This tool's interface, with visual “recipes” for data prep and the option to inject custom code when needed, makes it flexible: you can switch between coding and point-and-click as you see fit. This flexibility means analysts can start with simple drag-and-drop transformations and graduate to advanced Python or SQL within the same tool.
Pros:
- Collaborative & Easy: Offers a very friendly UI that simplifies building analytics projects end-to-end, reducing the learning curve for beginners and integrating well into various environments.
- Flexible Power: Provides a unique mix of no-code visual recipes and the ability to incorporate custom code (Python, R, SQL), boosting productivity for all skill levels.
- Rich Features: Includes built-in tools for data preparation with AI suggestions, machine learning algorithms, visualization, and essential collaboration features like version control and documentation.
- Enterprise Ready: Scales effectively for big data workloads by integrating with engines like Spark and Hadoop, while offering robust security, governance, and the ability to push heavy jobs for performance.
Cons:
- High Cost: Licensing is frequently mentioned as a significant barrier, potentially limiting broader access for analysts within an organization.
- Big Data Performance: While capable, complex workflows processing very large datasets within the built-in engine can experience slowdowns, often necessitating external processing setup (like Spark).
- Advanced Complexity: Although basic usage is straightforward, leveraging the platform's most advanced features or extending it via APIs requires deeper technical knowledge and presents a learning curve.
Best Use Cases:
- Cross-Functional Teams: Ideal for enterprise data science teams where data scientists, engineers, and business analysts need to collaborate effectively on projects.
- Diverse Skill Integration: Enables projects where varying technical skills are needed, such as analysts using visual tools while data scientists embed complex code within the same workflow.
- Unified Analytics Projects: Suitable for end-to-end use cases like customer analytics, supply chain forecasting, or fraud detection, especially when integrating diverse data sources.
Best AI Data Analytics Tool for Automating Data Prep
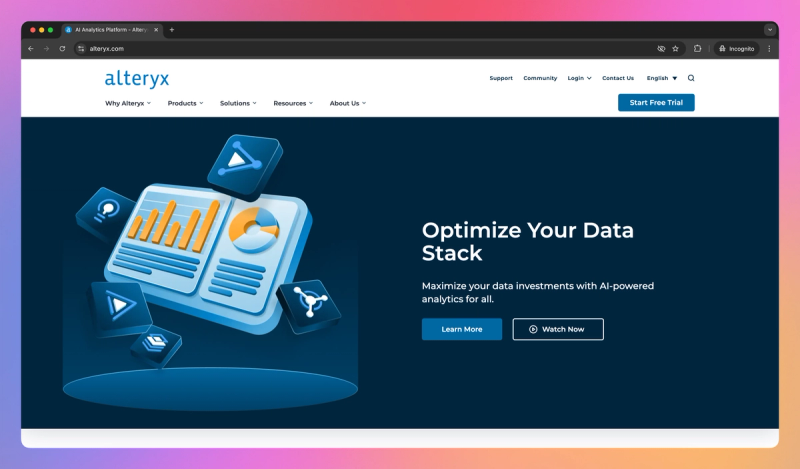
Alteryx
Alteryx is a veteran platform known for enabling advanced data preparation, blending, and analytics through a drag-and-drop interface. Its core value proposition is making powerful data tasks accessible without requiring you to write code. The Designer provides a canvas where you build workflows by connecting tools for data input, transformation, modeling, and output, dramatically speeding up tasks that might otherwise be tedious in spreadsheets or demand coding. This visual approach allows easy exploration and manipulation of data without complex queries. The platform particularly shines in automating repetitive processes; once a workflow is built, it can be run on demand or scheduled, freeing you from manual data wrangling.
Pros:
- Ease of Use: The visual workflow interface allows for quick onboarding, enabling you to perform complex tasks like joins or regressions using drag-and-drop tools without needing deep technical expertise.
- Powerful Automation: Automate tedious data preparation and ETL tasks easily, blending and analyzing data from multiple sources without writing code. Scheduled workflows significantly boost productivity by saving considerable time.
- Broad Integration: Connect seamlessly to databases, APIs, cloud applications, and popular BI tools like Tableau. While primarily no-code, the platform also allows incorporating Python or R scripts for advanced customization, offering flexibility across skill levels.
- Strong Community: Benefit from a large, active community sharing best practices and solutions, alongside reliable company support recognized by industry analysts.
Cons:
- High Cost: The licensing cost is a significant barrier, particularly for smaller teams or wider organizational adoption, as advanced features often require higher-tier plans.
- Big Data Performance: While efficient for moderate datasets, performance can degrade with extremely large datasets (millions of rows), potentially requiring workarounds like leveraging Alteryx Server or database pushdown.
- Advanced Learning Curve: While basic workflows are straightforward, mastering the full suite of tools and effectively debugging highly complex workflows requires a significant time investment.
Best Use Cases:
- Automating Complex Reporting: Ideal for automating time-consuming reporting processes that involve blending data from various sources, common in finance, marketing, or supply chain analysis, freeing up significant analyst time.
- Data Blending and Preparation: Quickly blend, cleanse, and prepare large, disparate datasets for analysis or visualization in other tools like Tableau or Power BI, especially when manual spreadsheet work becomes unmanageable.
- Citizen Data Science Tasks: Enable business analysts and citizen data scientists to perform advanced analytics, including predictive modeling and spatial analysis, using a code-free, drag-and-drop environment.
Best AI Data Analytics Tool for Searching Your Data Instantly
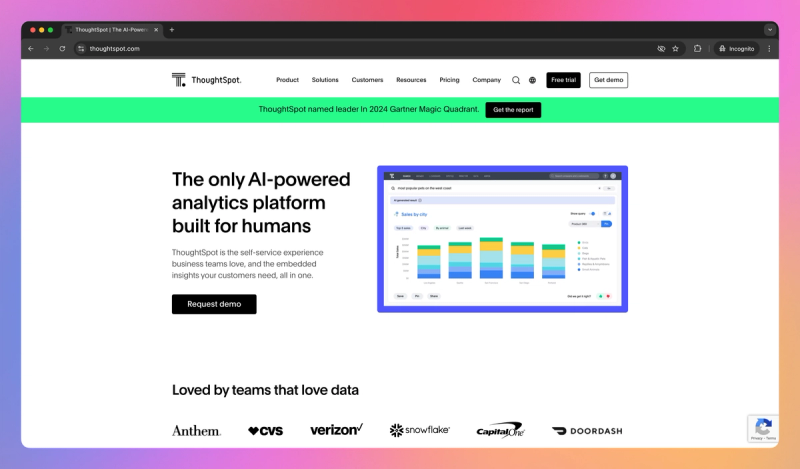
ThoughtSpot
ThoughtSpot revolutionizes business intelligence by allowing you to simply search your data in natural language and get instant results. Its core idea is “true self-service analytics” – even non-technical roles (like executives or sales managers) can type questions (e.g. “Total sales in Europe last quarter by product”) and the tool's search engine builds the query and returns interactive charts. This Google-like experience for data is hugely valuable for organizations drowning in reports; it empowers ad hoc exploration without needing a BI specialist. This platform also leverages AI to provide automated insights (“SpotIQ” insights) that highlight anomalies or trends without being asked, acting as an AI assistant for data analysis.
Pros:
- Search Interface: Its natural language search dramatically lowers the barrier to entry. You can ask questions and get answers, empowering non-analysts to self-serve insights and increasing data-driven decisions.
- Speed and Interactivity: Powered by a fast in-memory engine, the system delivers query results on large datasets in seconds, encouraging exploration through a responsive and agile analysis experience.
- AI-Driven Insights: The SpotIQ feature automatically finds outliers and correlations, acting like an AI assistant analyzing data in the background and providing valuable insights with minimal effort.
Cons:
- Data Modeling Prerequisite: The tool works best on a clean, well-structured data model. Setting up the ideal logical view can require significant upfront effort by data engineers to ensure the search understands data relationships correctly.
- Advanced Feature Learning: While basic search is easy, mastering more complex features like custom formulas or drill paths requires dedicated learning, potentially posing a challenge for traditional BI developers adapting to ThoughtSpot's approach.
- Enterprise Cost: This platform is an enterprise-grade tool with a corresponding price tag, potentially making it a significant investment, especially for smaller organizations.
Best Use Cases:
- Ad Hoc Exploration: Ideal for drilling into changing metrics and exploring hypotheses on the fly without waiting for data teams.
- Self-Service Reporting: Empowers executives and business users to get quick answers from data independently, moving beyond static reports.
Best AI Data Analytics Tool for Collaborating on Analytics Notebooks
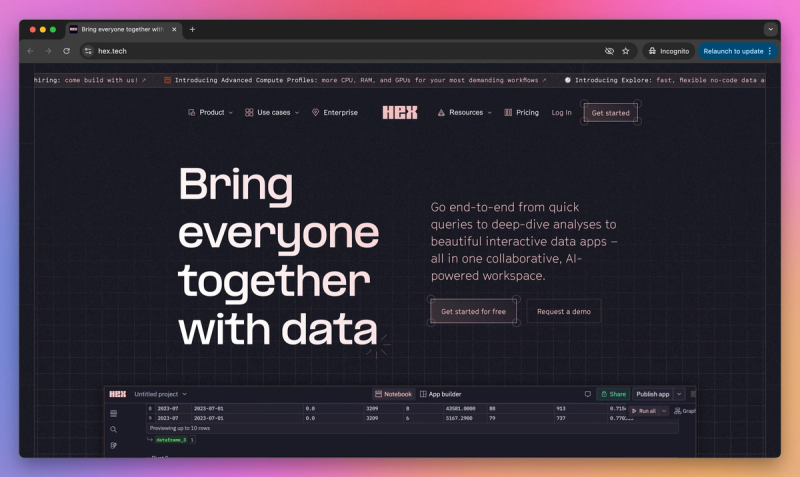
Hex
Hex is a modern analytics workspace that brings SQL, Python, and visualization together in one collaborative environment. It’s like a next-gen Jupyter notebook in the cloud, supercharged for teams. Hex’s core value is enabling data teams to go end-to-end: from writing SQL or Python to analyze data, to visualizing results, to sharing interactive data apps – all in one tool. This eliminates friction of switching between IDEs, BI tools, and notebooks. Moreover, this platform has built-in AI features to assist with code and queries, making analytics development even faster. The collaborative aspect (multiple users editing, commenting) and easy publishing of results as sharable apps are at the heart of its value proposition.
Pros:
- Unified Workflow: Combine SQL, Python, and visualization seamlessly in one place, significantly boosting productivity by eliminating context switching.
- Interactive Sharing: Easily transform analyses into interactive web applications for stakeholders, bridging the gap between technical work and business consumption without needing separate BI tools.
- Team Collaboration: Work together in real-time on projects with features like co-editing, commenting, and version control, improving teamwork and knowledge sharing compared to traditional notebooks.
Cons:
- Performance Bottlenecks: You may experience lag or performance issues when handling extremely large datasets or overly complex single notebooks, requiring workarounds like offloading processing.
- Scaling Costs: While a free tier exists, scaling up with more users on enterprise plans can become expensive, necessitating a clear ROI justification.
- Coding Prerequisite: The full power is unlocked with SQL/Python knowledge; while some no-code elements exist, it's primarily designed for code-comfortable analysts and data scientists.
Best Use Cases:
- Exploratory Analysis: Rapidly iterate on data exploration using SQL and Python in a flexible notebook interface.
- Internal Tools & Reporting: Quickly build and share interactive mini-dashboards or data-driven reports for internal stakeholders.
- Prototyping Models: Develop and test data models or analytical approaches before committing to full production deployment.
Best AI Data Analytics Tool for Automating Data Management
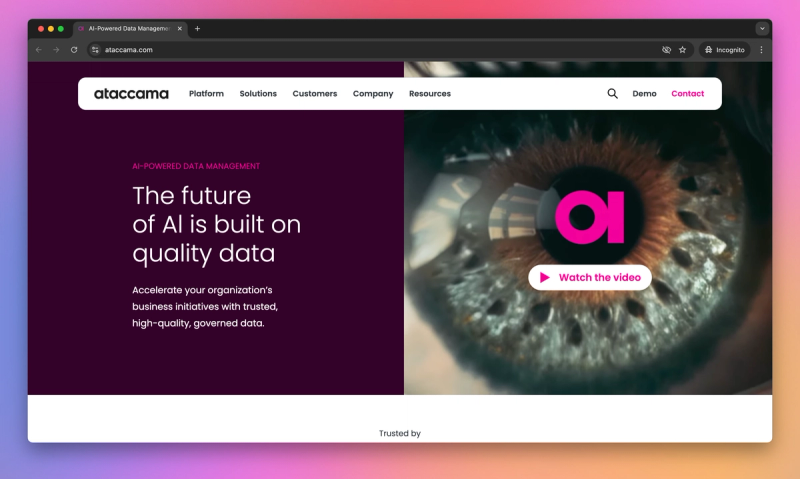
Ataccama One
Ataccama One is an all-in-one platform addressing data quality, master data management (MDM), data cataloging, and governance through automation and AI. It enables organizations to trust their data by automatically discovering, cleaning, and monitoring it at scale, unifying capabilities previously requiring separate tools. This platform uses machine learning to detect data anomalies, suggest data quality rules, and even auto-correct issues, breaking down silos between IT and data stewards for accurate, documented, and compliant data. Ultimately, this approach delivers more reliable analytics and simplifies regulatory compliance with less manual effort.
Pros:
- Unified Platform: You get data profiling, quality, MDM, and cataloging integrated into one solution, eliminating the need to juggle multiple tools and streamlining data management workflows.
- Intelligent Automation: The platform leverages AI for automated data cleansing, matching duplicate records, and suggesting quality rules, significantly reducing manual effort and speeding up data quality improvements.
- Robust Governance: It offers strong features for enforcing data standards, continuously monitoring quality, and providing enterprise-grade audit trails and workflows crucial for compliance.
Cons:
- Significant Complexity: Mastering the platform takes time and often requires specific training due to its comprehensive feature set; it's not a simple plug-and-play tool.
- Rapid Evolution: While innovation is ongoing, frequent updates and new features might mean some modules are less polished initially or require adapting to changes.
- Coding Sometimes Required: While aiming for low-code, implementing highly complex business rules or sophisticated transformations may still necessitate scripting or technical expertise.
Best Use Cases
- Enterprise Data Governance: Ideal for large organizations, especially in regulated industries like finance or healthcare, needing to implement comprehensive data quality, cataloging, and governance programs to ensure compliance and trust.
- Master Data Management (MDM): Effectively creates and manages single 'golden records' for critical entities like customers or products by profiling, cleansing, and merging data across complex system landscapes.
Best AI Data Analytics Tool for Automating Data Labeling
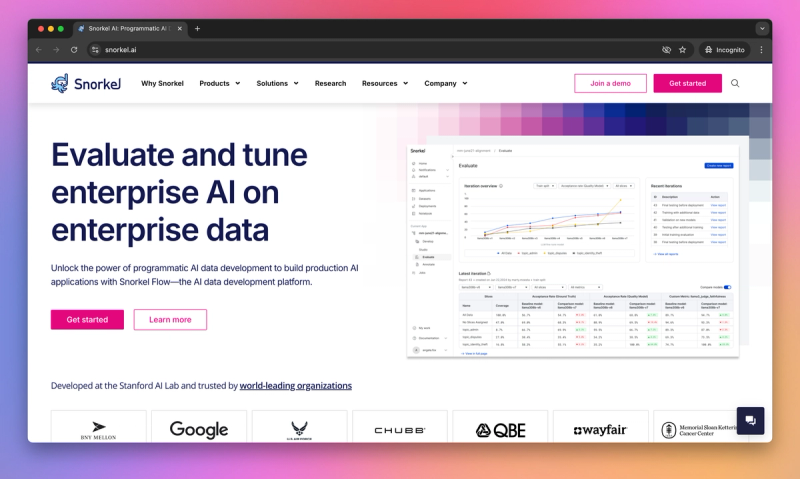
Snorkel AI
Snorkel AI's platform flips the script on traditional model development by focusing on efficiently labeling and developing the training data rather than primarily tweaking models. Its core value lies in enabling teams to build high-quality AI models significantly faster using programmatic labeling, weak supervision, and integrated model training in a loop. For many enterprises, obtaining large labeled datasets is the primary bottleneck; this tool provides a way to label data using code and rules (labeling functions) and train models with those labels, bypassing extensive manual labeling and making previously data-intensive AI projects feasible.
Pros:
- Rapid Development: You can dramatically accelerate model building, cutting project timelines from months to weeks by minimizing manual labeling. Writing a few functions can label thousands of items swiftly, especially useful when expert time is limited.
- Leverages Domain Expertise: The platform allows subject matter experts to encode their knowledge through labeling functions without needing deep ML skills, incorporating valuable business logic directly into the model pipeline for potentially more nuanced results.
- Iterative Improvement: By focusing on analyzing model errors and refining labeling functions where the model struggles, you can systematically improve model accuracy and generalization, often with less data than traditional methods require.
- Integrated Platform: Snorkel Flow offers a unified environment for labeling, data augmentation, training, evaluation, and error analysis, streamlining the workflow and eliminating the need to juggle separate tools.
Cons:
- Learning Curve: Adopting this approach requires your team to learn the distinct skill of writing effective labeling functions; it's a different paradigm than standard model tuning and takes iteration to master.
- Niche Focus: This tool is highly specialized for preparing training data for machine learning models (particularly classification and structured prediction) and isn't suited for general business intelligence or data analysis tasks.
- Setup Complexity: Integrating Snorkel Flow into an enterprise environment involves connecting it to data sources, which can require IT effort, especially with sensitive data. Convincing stakeholders to trust models built via weak supervision might also require internal education.
- Enterprise Cost: As a sophisticated enterprise platform, Snorkel Flow represents a significant investment that may be prohibitive for smaller teams or organizations not seeing a clear ROI from accelerated ML development.
Best Use Cases:
- Custom Model Development: Ideal when you need custom AI models but lack large, manually labeled datasets, particularly for text classification or information extraction tasks.
- Expert-Driven Domains: Highly effective in fields like finance, healthcare, legal, and government where data is plentiful but labeling requires specialized, expensive expertise or faces privacy constraints.
- Rapid Prototyping & Iteration: Useful for quickly assessing the feasibility of an ML task by building an initial model fast, and for maintaining models by updating labeling functions based on performance drift.
Best AI Data Analytics Tool for Building Business Predictions
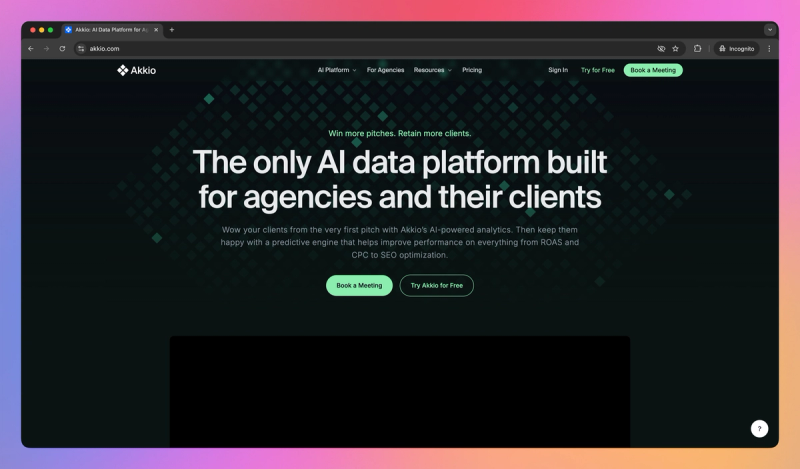
Akkio
Akkio is a lightweight AI analytics tool enabling the creation of predictive models and insights without coding or ML expertise. Its primary value lies in simplicity and speed, allowing business analysts or non-technical individuals to upload data (like CSVs or connected SaaS apps) and rapidly receive AI-powered predictions or analyses. The platform automates model training, evaluation, and deployment after you select a target variable. This accessibility brings automated machine learning to those lacking dedicated data science resources, facilitating quicker data-driven decision-making, such as predicting customer churn or lead conversion likelihood.
Pros:
- Exceptional Ease of Use: You don't need programming skills; the interface is straightforward, allowing model setup in minutes. This broadens AI accessibility significantly.
- Rapid Workflow: The entire process, from data import to deploying predictions, is streamlined, enabling fast experimentation with data-driven ideas.
- Actionable Integration: Connecting to tools like Salesforce or Snowflake and deploying results back into business applications bridges the gap between analysis and action, especially valuable for teams without dedicated engineers.
Cons:
- Potential Accuracy Limits: While easy, the automated approach might not achieve the absolute highest accuracy for highly complex problems compared to manual tuning by experts.
- Limited Expert Customization: The platform intentionally hides machine learning complexity, meaning you cannot select specific algorithms or fine-tune hyperparameters if desired.
- Focus on Tabular Data: Its strength lies with structured, tabular business data; it's less suited for unstructured data like images or complex NLP tasks beyond basic feature tables.
Best Use Cases:
- Sales & Marketing Predictions: Quickly build models for lead scoring, predicting customer LTV or churn likelihood, connecting directly to CRM data.
- Operational Forecasting: Use historical data for demand forecasting for products or predicting donor behavior for nonprofits.
- Rapid Prototyping: Agencies or small teams can prototype AI features for clients or internal use without needing a full data science team.
Best AI Data Analytics Tool for Unlocking Revenue Intelligence
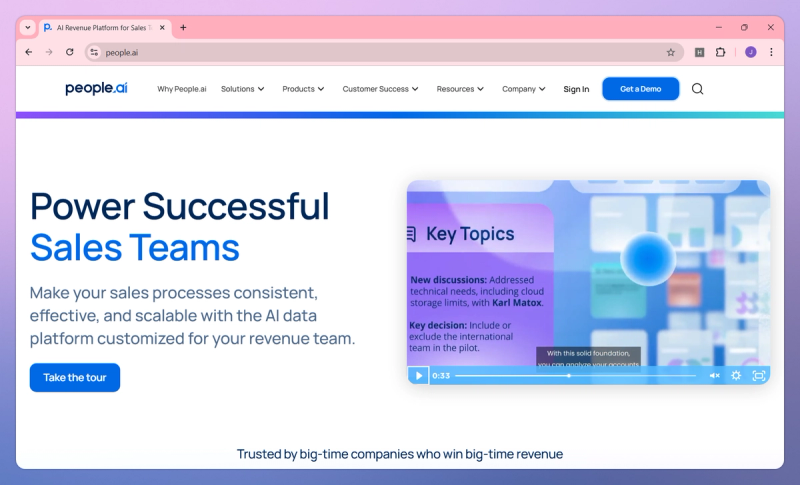
People.ai
People.ai automatically captures sales activity data like emails, meetings, and calls, analyzing it to provide insights that help sales teams drive more revenue. This platform turns the vast amount of sales interactions into actionable, data-driven coaching and forecasting insights by integrating with systems like your CRM, email, and calendars. It eliminates manual logging for sales reps, ensuring all customer touchpoints are recorded, and uses AI to identify at-risk deals, successful activities, and areas for salesperson improvement, offering real-time updates on performance and progress.
Pros:
- Automatic Data Capture: You save significant time as sales activities are logged automatically without manual effort, allowing your team to focus more on selling.
- Enhanced Visibility: Gain clear insight into sales execution with real-time performance data, enabling timely, data-driven coaching based on rep activity patterns and engagement levels.
- Improved CRM Data: Ensure your CRM is always complete and accurate with automatically captured contacts and activities, benefiting both sales reporting and marketing efforts.
Cons:
- Sync Delays: You might experience delays of a day or two for certain data, like call logs, to fully sync and appear in reports.
- Privacy & Change Management: Implementing the tool requires addressing potential privacy concerns regarding communication capture and managing the team's adoption.
- Cost Justification: The investment may be harder to justify for very small sales teams unless the productivity gains and insight value provide clear ROI.
Best Use Cases:
- Sales Activity Tracking & Coaching: Analyze rep engagement patterns, compare activities against top performers, and provide targeted coaching.
- Pipeline Review & Deal Qualification: Augment forecast calls with data-driven insights on deal engagement and risk levels.
- Onboarding New Sales Reps: Accelerate ramp-up time by showing new hires what successful activity levels and engagement patterns look like.
Conclusion
Modern AI analytics tools are transforming how organizations work with data by automating tedious tasks and surfacing insights that were previously hidden. These platforms make advanced analytics accessible to users across technical skill levels while enabling faster, data-driven decisions. The result is more efficient teams that can focus on strategic work rather than manual data wrangling.